인터랙티브 데이터 마이닝을 가능하게 하기 위한 마이닝 모델들의 선택적 저장
The present invention refers to data mining models (data mining models) are computerized method for storing, data processing systems, and computer program number relative to with article. Data mining (data mining) generally relates to hidden data for extracting information from input data (data a-driven approaches) has a center approaches - age. In addition method for splitting and conform depends upon the types of information data are extracted data mining model vehicle from the outside. Additional process information against information model further analyzed or verified substrate. Data mining technique effectively mass data processing method relate generally to consider the flow tides. First example number defect/deficiency transmitted account number numerical control machine. Wherein, the input data is the origin of components and features can be associated with a plural number of data. The aim of the quality analysis and quality assurance (quality assurance) number associated with data mining in context bath door number solution may be disclosed. Data mining is, e.g., for root cause analysis, number bath within a facility for early warning systems, and to reduce the claim for quality assurance, can be used. Second example, considering defect/deficiency various information technology system, wherein, data mining is intrusion detection, monitoring system, and door number can be further used for the analysis. Data mining is in addition, e.g., typically it is big [su[su] [me[me] behavior can be analyzed tail (retail) and services in, and mined in clinical studies for causality in medical and life science, has various other examples. Data mining general outline, URL http://publib. Boulder. Ibm. Com/infocenter/db21uw/v9r 5/index. Jsp?Topic=/ com. Ibm. Dwe. Welcome. Doc/dwev9welcome. In IBM InfoSphere htmlTM Warehouse information in (Warehouse information), the URL http://www. Redbooks. Ibm. Com/abstracts/sg247418. In IBM html (Redbooks) red books so as "Dynamic Warehousing: Data Mining Made Easy" of 1 field (pp. 1 - 8), 2 field (pp. 9 - 26), 4 field (pp. 39 - 50), 7 field, section 1 - 3 (pp. 139 - 175), and RF antenna A (pp. 331 - 366) encoded in ball number. These documents are data mining of three discovery method, i.e., clustering (clustering), associated rules (association rules), and sequences (sequences) covering the substrate. Clustering data mining (clustering data mining) makes it possible to separate clusters or groups of data records having attributes slightest to find a match. An allogenic (homogeneous) of one cluster records are then must then, clusters of records are two different heterologous (heterogeneous) should then as much as possible. Any items in transactions (transactions) association rules are requested (describe) techniques whether the patterns are disclosed. Data mining sequences (sequences data mining) input data items in the exemplary time-ordered given sequences (time a-order sequences) arranged finding other. Traditional data mining scenario are mounted to one of the user data mining work be run at design computed assuming that the other. Such as data mining processes may involve repetitive CRISP provided DM (Cross Industry Standard Process for Data Mining) designed to allow such scenarios. These process are typically as background work carried out off-line. In order more detailed content, URL http://www. Crisp-a dm. Org can be referencing. However, many current and future data mining scenarios rather is at or other features. I.e., first, data mining plan work but, during interactive analysis process called ad-hoc (ad hoc) (interactive analytical process) are disclosed. Second, data mining is partially overlapping tasks having the same data gathering reduces many different users (datasets) (in parallel) invoked by a substrate. A significantly higher response than interactive data mining (interactive data mining) offline data mining may request recovery are disclosed. This space that is analyzed data in severe cases in particular door number gathering (datasets) are disclosed. If follows that each user data mining independently of each other, can be case is wasted resources. One method to solve this door number for the purpose of cache algorithm generic data mining models are disclosed. The, if data mining model is configured, data mining model is one issue a query be returned as well as user, even cache overnight. Then, some users share the same cache model to other. If another user has already if the configuration model, it again later in the rebuild (rebuild) WD other. However, the users may specify various parameters can be storage space for storing all data mining models (built) number one is a build-is encoded. Database in fields, semantic caches (semantic caches) wheel developed, these queries knows how related each other, so that it enables a more intelligent caching strategy (intelligent caching strategy) information for plural times. S detailed content is translated. Dar, M. J. Franklin, B. T. Jonsson, D. Srivastava, and M. Tan: "semantic data caching and replacement (Semantic data caching and replacement)", (Proceedings of the international conference on Very Large Data Bases) Conference event are very large database relating to station number (1996, page 330 - - 341) thereof can found in. These database query the caches are data mining models support caching (caching) is not suited for disclosed. In the context of data mining association rules, caching techniques to be known. B. Nag, P. Deshpande, and D. DeWitt, "multi-dimensional data mining queries (Caching For Multi-a Dimensional Data Mining Queries)-time", cis reel mix (Systemics), and a station number information every tic [su[su]between the tic [su[su] which it earns (Cybernetics) (Informatics) (SCI-a 01) Conference event are (2001, this year column, Florida) is, semantic information (semantic information) according to the results of association rules mining queries a number storing chunk-based cache (chunk a-based cache) into each other. But the item set number encoded into one method. T. Morzy, M. Wojciechowski, M. Zakrzewicz, "implemented using fast sequential patterns of discovery (Fast Discovery of Sequential Patterns Using Materialized Data Mining Views) data mining views", computer and information science relating to 15 difference station number core Forge bud (ICSIS) Conference (2000) are implemented of rows of, i.e. cached data is mining queries of data mining views a new data mining queries are mapping method for clinical use other. These approaches in addition to the encoded number one mining association rules. Existing approaches said any models cache 31b response frequencies can be implemented effectively resolve the question as to whether how additional reduction the tropics. Thus, the purpose of the invention is for use in data mining models if it became grudge number communicated to storage space for storing a computerized method, data processing system, and computer program number number under public affairs article are disclosed. Side data mining models of the present invention number 1 for storing a computerized number 1 method number under public affairs substrate. Comprising the number 1 method includes the following steps. I.e., number 1 the data mining model is generated, said number 1 data mining model includes the following features, i.e., at least one quality (quality) and complexity (complexity). If said reference features data mining model storage means for storing said number 1 is met candidate handled with each other. Said number 1 additional data mining models already stored on said storage means data mining size and storage limit exceeds the sum of the sizes (storage limit) whether a determination is carried out. If said storage limit is not exceeded, said number 1 data mining model is stored in said storage means. If said storage limit is exceeded, said number 1 data mining model and said additional data mining models to which data mining models based on priorities of said storage means store the estimation of the data is given to a vehicle from the outside. Said at least said priority can include each data mining models to access frequency depend on other. Data mining requests for data mining basis from transfer (surjective) mapping and data mining models from data mining tasks (N - to -1) shear transfer (bijective) (1 - to -1) mapping is 1308. ball number. Data mining work has an associated data mining model may have. Each stored data mining model, each work technique (task description) can be stored. As soon as it receives a request for a new data mining defect/deficiency, said transfer and said storage means based on said new data mining request said shear transfer mapping already stored can be a determination is made as if it would be worthwhile. Data mining model - associated with said new data mining request after processing (post-a processing) can be performed. Said number 1 data mining model, although said number 1 data mining model for use without additional stored information identifying said number 1 data mining model and said number 1 data mining model features (describe) described information can be stored. Data mining model is a set of data patterns (set) can be. The data pattern is determined by has an associated pattern characteristic of said data mining work may have. Said data mining model based on said pattern data mining quality is can be calculated. Said data mining model complexity build time (build time) can be determined based on said data mining model. The next priority is data mining model features, i.e., to access frequency (access frequency), storage size, build time, and quality can be calculated based on at least one. Said storage means, based on the previously stored in the data mining models are 1308. the wetting ability of capabilities of their number. Said fins (data selection constraints) lines (data mining constraints) to transfer the mappings are data selection number and data mining number based on the data mining requests comprising comparing data mining tasks can be. For storage of the present invention number 2 side data mining models a computerized number 2 method number under public affairs substrate. Comprising the number 2 method includes the following steps. I.e., for data mining models, information identifying the data mining model is stored in storage means contains information that describes and data mining model quality. Performing data mining model number 1 pre-defined criteria quality data mining models are stored in said storage means. As soon as it receives a request for a new data mining defect/deficiency, new data mining is not said data mining model is determined, said data mining for a model to data mining model is stored in said storage means contains information that describes a quality certified check carried out. If said data mining model data mining quality of a predefined criterion number 2 if, a user may desire mining cost and the maintenance cost are request portion and a second portion. Data mining model is can be comprising the union of data patterns. The data pattern is determined by has an associated pattern characteristic of said data mining work may have. Said data mining model based on said pattern data mining quality is can be calculated. For storing data processing system of the present invention number 3 side data mining models number under public affairs substrate. Said system includes a data mining models (storage means) for storing comprises storage means and data processing means (data processing means). Said data processing means are employed to generate the data mining model number 1, said number 1 data mining model includes the following features, i.e., at least one quality and complexity. If said features reference is met, said data processing means is said data mining model storage means for storing said number 1 candidate handled with each other. Said data processing means is said number 1 additional data mining models previously stored in said storage means data mining size and storage limit exceeds determines whether the sum of the sizes (storage limit). If said storage limit is not exceeded, said data processing means is said data mining model storage means store said number 1. If said storage limit is exceeded, said data processing means is said number 1 data mining model and said additional data mining models to which data mining models based on said storage means whether a store. Said at least said access frequency characteristics are each data mining model depend on each other. For storing data processing system of the present invention number 4 side data mining models number under public affairs substrate. Said system includes a storage means, input means, data processing means, and output means. Said storage means is mining models for data mining model stores information identifying the quality and data mining model contains information that describes, storage means store said data mining models. Said input device includes a new data mining request d2. Said data processing means and data mining model quality information identifying the data mining model store information describing said storage means. Said data processing means is of a predefined criterion number 1 data mining model storage means stores said quality data mining models. Said data processing means, said data mining model is not determining new data mining in addition said data mining model data mining model is stored in said storage means contains information that describes quality has been check. If said data mining model number 2 of a predefined data mining model quality which does not access approval criteria if, said data processing means is provided with a first surface mining for users to make sure that the disclosure request to each other. Said outputting means output said request said user to other BTSs. For storing a computer program of the present invention number 5 side data mining model number article (computer program product) number under public affairs substrate. Said computer program product including a computer usable medium number (computer usable medium) used, said computer usable medium having computer usable program code (computer usable program code) implemented therein. Said program code to perform the steps according to one of the present invention number 1 and number 2 sides speed to consists of. The present invention for a better understanding how the present invention can be carried out effectively whether, the number appended drawing method of reference as reference example along only are disclosed. Figure 1 shows a flow scheme of the present invention also one in the embodiment according to data mining models of number 1 method for storing when etched. According to one embodiment of the present invention to data mining models 2 and 3 is also for storing number 2 method shown flow of degrees to each other. Figure 4 shows a clustering data mining model when a one example of the present invention also one in the embodiment according to for storing etched. 5 to 7 of the present invention is also one in the embodiment according to data mining models describing flow selective and interactive storage shown substrate. 8 to 10 of the present invention is also one in the embodiment according to data mining models association rules for storing sample scenario illustrates the substrate. Figure 11 shows a number 1 of the present invention also one in the embodiment according to data mining models in a data processing system for storing block decodes in degree. Figure 12 shows a block of data processing system of the present invention for storing data mining models also one in the embodiment according to number 2 etched in degree. Data mining model is based on data mining work limited thereto. Data mining work (task description) type data mining techniques, data mining is applicable to the data lines for data selection number (data selection constraints), and said data mining dependent data mining number lines (data mining constraints) to specify other. Data mining request results in a call number by data mining work chamber are disclosed. Said corresponding data mining work request transfer (surjective) - to - (N - to -1) mapping one (many-a to a-one) etc. parameters comprising the. Data mining operations are associated with a different transfer the mappings are data mining request to other (although said requests and a task number lines and other other parameters even though having the different data mining). One - to - shear yarn (bijective) one (one-a to a-one) (1 - -1 to) the mappings are associated with data mining work such as returning data mining model. Said mapping are performed based on the program code portions and configuration parameters (configuration parameters) can be. According to of the present invention in the embodiment for storing said data mining models are selectively semantic cache data mining estimation considering other. Caching (cashing) or implementing portions of the temporary memory (materialization) or permanent storage device (e.g., hard disk) storing information for use further portions of data mining models by big. When said requested cached information again, it allows the user to be returned be faster. However recalculation (re-a compute) said data mining model information does not need to be's oldest. Said user request which can be direct response from the information in the cache (cache hit rate) percentage of hit bind potassium channels of vehicle from the outside. Data mining models when requested, the contents of said cache re-calculated priorities (dynamically) to dynamically based on updated, recalculated at least said data mining models based on priority access frequency limited thereto. This calculated which make it difficult and frequently used data mining models in view of short response times and, said memory or other storage devices consume space does not result in the activating step. Figure 1 shows a flow of data mining models also for storing also number 1 method (100) when a is etched. In step 101, data mining model number 1 generated, scrap features, i.e. at least one quality and complexity. In step 102, whether the criteria are met for said features testing is carried out. If no if, processing is completed at a time t5. If for example if, data mining model storage means for storing said number 1 in step 103 candidate to handling with each other. In step 104, data mining said number 1 said additional data mining models already stored on the storage means size and the sum of the determined size. In step 105, comes into the predefined storage limit is exceeded to whether testing is carried out. If said storage limit is not exceeded, step 107 said number 1 in data mining model is stored in said storage means. If said storage limit is exceeded, step 106 said number 1 in data mining model and said additional data mining models to which data mining models based on characteristics of said storage means store the determined whether. Said at least said access frequency characteristics are each data mining models depend on each other. Figure 2 shows a data mining models also for storing number 1 number 2 method of improving the flow (200) when a is etched. In number 2 method, step 201 as shown diagrammatically, and data mining model quality information identifying data mining model contains information that describes data mining models have been pre-generated for stored in storage means. Step 202 as shown diagrammatically, said been pre-generated data mining model includes a diode and a data mining model number 1 qualities of a predefined criterion whether test with each other. If for example if, step 203 as shown, these been pre-generated data mining models are stored in said storage means. Otherwise, they are is stored. Figure 3 shows a number 2 method also for storing data mining models by improving the flow of number 2 (300) when a is etched. Step 301 N. new data mining request. Said new data mining data mining model in step 302 is not determined. Step 303 contains information that describes this data mining model in data mining model quality of Figure 2 has been previously stored in the storage means is said check step 201. If step 303 back check is unsuccessful (fail) (no branch), said new data mining is not processing is completed at a time t5. If the check of step 303 success (e.g. branch), step 304 is of a predefined criterion whether said data mining model number 2 quality testing substrate. If step 304 if of test fails, said new data mining request is not further processed. If the success of test step 304, step 305 in the user data mining cost and the maintenance cost subjected request portion and a second portion. If the user confirms the in step 306, step 307 requests in said new data mining data mining is the processing advances. Otherwise, processing data mining request does not continue. Figure 4 shows a clustering also decodes a data mining model for storing in one example. Example said four data mining requests (411, 412, 413, and 414) which, each identifiers R1, R2, R3, R4 and a. Said data mining type always cluster are disclosed. Each request is said tables A, B, and C data selection one specify other. Said transfer mapping procedure said data mining type and said data selection number said copied data mining request to generate one data mining jobs, said corresponding data mining tasks can include said data mining tasks (421, 422, and 423) which is one, each identifiers T1, T2, T3 and has. One said request said number mapped to one data mining operation are as follows data mining number 2000. I.e., the user data mining model for calculating the maximum number of clusters can be displayed. For example, data mining request (412) in MaxNum=10 and data mining request (414) MaxNum=2 in are disclosed. The maximum number of data mining request is cluster MaxNum already existing data mining work without such as or higher number can be mapped. The maximum number of clusters does not displayed user, e.g., data mining requests (411 and 413) when in MaxNum=auto, said clustering data mining system is expected data mining type and data selection number previously stored in the data mining basis that such requests having one attempts to mapping. If this is not possible if, MaxNum predefined value for new work technique (task description) is overnight. Then, said data mining system is associated with a data mining said data mining model is defined. Maximum number of clusters in the user number 1 without being displayed, for example, by data mining request table A MaxNum=auto cluster of data (411) and to other BTSs. Said data mining system provides a novel data mining work request identifier for R1 T1 having number 1 (411) and mapping, data mining work for T1 and set to a value of a predefined maximum number of clusters MaxNum=10, assuming that the data mining model M1 associated with calculating defect/deficiency. R2 having request identifier number 2 (412) is expected table A and values of less than or low value MaxNum=10 specify other. Said data mining system is automatically determined parameters to said evaluated about physics that the thread number MaxNum=10. Then, said number 2 request is mapped to the same data mining work T1 are disclosed. Calculating a new data mining model number 2 request (R2) instead, be cached data mining model M1 be returned to a user. Data mining work T1 T1 data mining work that has the same data selection number one specify clustering data mining request is equal to or lower MaxNum value T1 can be mapped to data mining work. Therefore, the data mining model M1 T1 data mining work generating equalization or higher general data mining work can be taken into account as well. If clustering data mining request R5 is data mining model number number of chamber lower MaxNum clusters in M1 and thermally in addition data mining request R5 is mapped to data mining work if T1, data mining model for calculating the M1A post - processing to 10sup16. M1A is data mining request R5 accurately up to and including the specified number of clusters, said clusters and classifies the portion of data mining model M1 - after processing included in the substrate. If clustering data mining request R6 having the same data selection number one higher than existing data mining work MaxNum T1 has a second value, data mining request mapping R6 can be impossible. Then, the T6 said new data mining work value associated with the data mining model is populated with the requested MaxNum generating M6 build with each other. The new data mining request identifier R3 (413) in, the maximum number of clusters is not specified. I.e., MaxNun=auto are disclosed. The identifier T2 new requesting R3 new data mining work (422) mapped to the other. T1 data mining job is independent from work are disclosed. However said data selection other table rather than B are disclosed. Data mining work (422) the maximum number of clusters of predefined set to a value with each other. I.e., MaxNum=5 are disclosed. M2 is created wherein associated data mining model, this has 5 or more clusters. R4 identifier having additional new data mining request (414) data on selection table with C specify other. Said request identifier T3 having new data mining work (423) mapped to the other. T1 and T2 are independent from working operations are disclosed. However performed on another table C because data mining are disclosed. R4 identifier with request (414) is the maximum number of clusters being displayed as follows. I.e., MaxNum=2 and, clusters having the same maximum number is coupled identifier T3 work (423) mapped to the other. I.e. MaxNum=2 are disclosed. Data mining work (423) is associated with data mining model M3 is configured, it is data mining work (T3) specified, the maximum number of clusters, clusters may have smaller than MaxNum=2. (T1, T2, T3) associated with each data mining work (M1, M2, M3) to result in the execution of the data mining model to 2n.. Each data mining model is data mining model features set (431, 432, 433) with respect, this data mining model, quality, complexity, access count (access count) or access frequency (access frequency), comprising storage size information. All the characteristics are measurable disclosed. I.e., these are represented by a numerical value. For example, demographic clustering (demographic clustering) such as multiple data mining method and nose lake [neyn[neyn] neural clustering (Kohonen neural clustering) are known. For example which are two method provides a string models, each model comprises a separate number of clusters (discrete number of clusters). Said clustering model range [0.. 1] model at a quality number Q can be. Said demographic clustering model is a model of quality number Q (homogeneity) total of clusters in which symbolically, the coherency of the average similarity (mean similarity) said two cluster cluster record are disclosed. When ring nose lake [neyn[neyn] said cluster, said cluster said model quality number can be measured by the average distance between said sorted data records a best-matching cluster center when said cluster symbolically rates are disclosed. Said data mining model complexity build time (build time) determined based on said data mining model. This said data mining model for calculating the time (on the fly) the spot are disclosed. Said access count or time window (window) access frequency in data mining model portion of hits (fraction) are disclosed. Said cache storage amount to be consumed by said data mining model storage size are disclosed. Said temporary memory cache, or e.g., such as the hard disk permanent storage can be implemented. Said four data mining model characteristics any thread number instead of measured values, corresponding values in addition other characteristics (e.g., from said data mining work details (details)) can be evaluated based on. This evaluation data mining model quality evaluated, evaluated data mining model complexity, access count or evaluated evaluated access frequency, and data mining model number under public affairs size can be evaluated. Said determined data mining model features a decision regarding completion of storing said data mining model independently to information stored in said cache. Said data mining model based on characteristics, implementing reference (materialization criterion) can be evaluated. Further implementations store the complete data mining model for use. Said evaluation for each data mining model implementation state 2n. (materialization state). One of the values having three said implementing state variables (materialization state variable) may have. 1. ". ". "". "Materialize=YES" is, if too low quality data mining model without said data mining model build time is not too short, and not said cache storage limit from being exceeded, said calculated data mining model cannot be rerouted to another cache by a goniophotometer. 2. ". ". "". "Materialize=NO" is, if said data mining model build time is too short or too low quality said data mining model, not to the cache by said data mining model calculated by a goniophotometer. 3. ". ". "". "Materialize=MAYBE" is, said cache storage limit exceeded said data mining model quality without too low because even when said build time too short, data mining request number 1 after computation for data mining model exhibits do not stored value. In the change request, said cache said data mining model is a structure having sufficient free storage space can be recalculated, ultimately stored in said cache. Implementing said reference can be represented using the following formula. IF (ModelQuality < MinimumQuality OR ModelComplexity< MinimumComplexity) Materialize=NO ELSEIF (CacheStorageLimitNotExceeded) Materialize=YES ELSE Materialize=MAYBE ENDIF The following threshold values (i.e., MinimumQuality=0. 5 and MinimumComplexity=0. 3) in the case of, implementing state (442) for the computation of the model M2 Materialize=NO returns. However, its quality threshold level lower than said number 1, since its complexity threshold lower than said number 2 are disclosed. The reason is that smaller than said second minimum build model build time in another substrate. Said data mining model is the spot can be easily calculated, T2 is implemented model for a M2 does not work. Each models for implementation States (M1 and M3) (441 and 443) and MAYBE the Materialize=YES are disclosed. This has a storage cache exceeds said limit is already offered not be, otherwise dependent on the other. Therefore, model M1 work T1 are linked to said predefined threshold than higher quality and complexity and, since it does still exceeded embodied in said cache storage limit. In addition plastics when its access frequency is increased work T3 model M3 can be implemented. If YES or MAYBE implementation of state which is set model, said model priority is awarded and, this typically access count storage size and said data mining model size may depend on disclosed. Data mining models also 5 to 7 selective flow describing interactive storage shown substrate. Them also are connecting elements (connector elements) (1, 2, 3, 4, and 5) interlinked by (interlinke) with each other. Said first used as a data mining system, manager registered user (administrator user) is considered suitable for several constructs that are unique and cannot be estimated on the spot can be specify data mining tasks. These data mining task data mining models associated with said data mining system said pre - calculated (pre-a computation) and said cache for storing them for implementation the total family it will yell and it makes criteria check. As shown in fig. 5, in step 501, said data mining system is data mining request d2. In step 502, data mining request received said data mining system is equal (equivalent) data mining operation or said received said data mining request than general check whether said data mining operation in the cache. In the case of lowest likelihood and a second, said data mining request is received cached data mining work or higher in more specific than said limited number are disclosed. Data mining requests parameters and data mining jobs are represented by one number bonds (conjunctions). Said parameters may simply binary relationships can be characters including a (binary relations). For example, "10" and "Table A" DataSource=MaxNum=are disclosed. Then, step 502 is performed very effectively by a simple logical defining (entailment) can be. Such a data mining requests for data mining basis from transfer mapping, i.e., disclosed - to - one mapping can, request set of binary relationship between set of tasks are disclosed. The preferred embodiment is to determine relationships to chamber number two mechanisms 1335. Said first mechanism parameter specified is not attached to the request (request description) techniques, e.g., MaxNum=auto when received signals. Said data mining system is said data mining operations not automatically determines this pre-specified parameters. For example, MaxNum=10 are disclosed. Second mechanisms interposed between the inclusion of relationship (subsumption relation) number using substrate. When the request number to meet more specific one, it is more general number having one jobs can be mapped. For example, a data mining request is mining number having a data mining number MaxNum=2 mapped to data mining work can be populated with the MaxNum=10. However since a smooth second number first than general are disclosed. Existing data mining operation when said data mining request is successfully mapped, processing are continually over the connection element 1 in Figure 7. If step 502 received said data mining operation in any suitable data mining requests if a found in said cache, said data mining techniques (task description) in step 503 the system data mining request cache parameters can be received as said storing. Said work data mining techniques build (build) mapping between a shear yarn, i.e., one - to - one mapping is disclosed. Then, in step 504, data mining system said corresponding data mining model having different characteristics and data mining that make up the model, said cache storing said data mining model features can be. Said information from said data mining system is said data mining model works corresponding techniques can be updating is coupled. If said cache if said corresponding data mining estimation are already stored, they can be updated. Said data mining model features model identifier, model quality information, storage size, access can count and build times. Data mining work techniques (descriptions) and data mining model features, their own data mining models are not stored in said cache for said can be in the cache. In step 505, the data mining model quality lower than said data mining system said predefined minimum quality lower than said predefined minimum build time requested recipient or build-time check. If for example if, said build data mining model will not be embodied and, said process continues to step 508 are disclosed. When the result of the step 505 "no" (no), said data mining system is said data mining model build additional data mining models to those previously stored cache size and said determining the sum, the sum is testing whether predefined storage limit exceeds 2000. This if said cache if said stored data mining model build will be drained storage space means other. If not exceeded said storage limit, said data mining system stages in said cache store said data mining model build 507. In step 508, said data mining model allows the user to return and build, this independent from said cache storing said data mining model or otherwise disclosed. If in step 506 when said storage limit from being exceeded, processing are continually over the connection element 3 in Figure 6. In step 6, processing also 5 from connection element (3) through start with each other. In step 601, said data mining model and said said data mining system is previously stored in the data mining models build calculates priorities. Data mining priority is the following characteristics, i.e., data mining model storage size, its access count or to access frequency, its build time or to reduce the calculation time, and its quality can be determined based on at least one. Said data mining priority is said data mining model product (product) of the counts access said storage size implementation being. Said access count number corresponding data mining model under public affairs wherein user number of requests, this divided by the total number of user request can be described. Said access count or time window access frequency measurement is typically defined by a substrate. Preferably, said build data mining model is one obtain access frequency value. In step 602, the data mining system said, previously stored in the data mining models higher than priorities whether test as follows. If were not, said data mining model is build and lowest priority, said data mining system structure is stored in a cache to said determines. Processing in Figure 5 connection element (5) are continued through the. In step 508 said build data mining model returned to a user. Priority higher than the case when a previously stored in the data mining model, said model is encoded number from the cache in step 603 the wetting ability. Again, step 604 said data mining system is previously stored in the data mining model in data mining models build said size and said sum exceeds said predefined storage limit magnitudes determine test as follows. If, for example if, in processing step 602 previously stored in the data mining model for testing whether the lowest priority continues substrate. If said longer storage limit is not exceeded, said processing said cache for storing said data mining model build, step 507 5 to connection element (4) and the processing advances through. In step 502 of Figure 5, said data mining request said data mining request data mining work equivalent to or not less general than found in said cache, said data mining system is, in Figure 7 connection element 1 through continued and substrate. Said data mining model in data mining model quality associated with said data mining system step 701 a minimum quality threshold lower than a testing substrate. If said quality is too low, and issue warnings to a user for said data mining system is in step 704, and a second portion said data mining cost and the maintenance cost to ask users to other. In step 705, said determining that said user can not use of the model, then said data mining request processing of the completed at a time t5. It is judged whether the data mining in step 705 such that said user, said data mining system includes a connection element (2) continued through the with each other. Again in Figure 5, in step 504, data mining system said determined at step 502 data mining task associated with data mining model build other. In step 701 a minimum quality threshold higher than said data mining quality when said, said data mining system is associated with said data mining model in step 702 whether said cache testing substrate. If were not, said data mining system is in connection element 2 and continued through the step 504 of Figure 5, associated with said data mining model build other. If said associated data mining model to be stored in said cache, said data mining system is associated in step 703 - processing on said data mining model after can be. Data mining request when said corresponding data mining work equal, - can be any after processing is required, said step in said cached data mining model is user be returned directly be 508 of Figure 5. Said data mining request corresponding said data mining operation than in normal situations, the data mining request cached said sub - model (sub-a model) can be generated from data mining model. Connection element (5) through, said user in step 508 - model is be returned be presents of Figure 5. Model of said sub - depends upon the types of data mining can be. In the case of clustering data mining, cached data mining model number of clusters can be reduced by the overall existing clusters (aggregation). For mining association rules (association rule mining), practical examples provided hereinafter described in. Data mining is performed essentially corresponding first data set is (essentially), said altered data based on a set of said data mining models are all encoded number from the cache the wetting ability. However, access count and quality information about said cache can be still maintained. These properties are fulfilled data mining method applied depends on the nature of said selected data itself are expected. However, said data mining model build time and storage size accomplishing that take into account the amount of data increases. 8 to 10 for storing sample scenario data mining models association rules is also shown other. Any items in transactions associated rules describing whether a frequent occurrence patterns are disclosed. Collection of items (set) I={I1 , I2 , .. , Im } Defect/deficiency taking into account. D set of transactions is a unit length, wherein each transaction T is belonging to the subset of items I (subset) are disclosed. Transaction T has a subset of items from the I A={I1 , I2 , .. , Ip } And include, in addition I items from the additional subset B={Ip , Ip+ 1 , .. , Iq } Can be comprising, A and B are each bovine (disjunct) subset subset are disclosed. I.e. are also do not have any common elements. In the form of partially set between the subset A B A association rules → B, i.e. {I1 , I2 , .. , Ip } → {Ip , Ip+ 1 , .. , Iq } Selections of meanings (implication) are disclosed. A subset of items includes a body (body) and bind potassium channels, said subset of items is head of rules (head) B bind potassium channels of vehicle from the outside. All possible from the collection rules for selecting a program of interest rules (interesting rules) to, important and various measures of interest can be a number one (of significance and interest) are used. (Support) and reliability (confidence) support a best-known number one are also a threshold minimum inclusion content. Item set is a data set including A supp (support) (A) also support A item set of transactions at portions defined as. A rule → The reliability of the conf B (A → B)=supp/supp (S) defined by (A), wherein T is set in subset B A subset S D transactions in transactions and items of the union (union set) are disclosed. This, if c % of transactions in a subset of items in addition including D A B subset of items is a if so, A association rules → (C) maintain true D B loads transactions with a set (true) means that other. In other words, said trusted (conditional probability) conditional probability, P (B/A), i.e. a transaction T subset A occurs in under an assumption that, in subsets B probability transaction T are disclosed. Some applications may be permitted to be loose or very rare (loosely) since it is not as important may be correlating events and then, the user can also or reliability of said rules defining the minimum support. An example of a frequently then described. Said Company has large licensed dealer network (large licensed dealer network). Said repair shop services (repair shop services) in addition to the dealer number under public affairs substrate. Sales (sales) and deficiency (defects) are data that relates to said integrated transferred regularly or frequently (regularly). Different people having different work serves regularly said data analysis operation and is coupled a plurality of hierarchies. This work role among people or ensure quality standards and for supporting said dealer. These call data including the central data warehouse for analysis requests (against) can be disclosed. Are the same or similar analyses often call request as follows. Therefore, number of times the cached response analysis results can be reduced significantly, the productivity can be increased. Implementing reference, i.e. said data mining model said cache when said determining procedure recording the cache storage limit, said data mining model quality, and said data mining model based on an elapsed time and build, is been defined above. Said data mining system for said a systems administrator next parameters, i.e., CacheStorageLimit=9 MB, MinimumQuality=0. 5, and specify his MinimumBuildTime=10 minutes. (R1A, R1B, R2, R3A, R3B and) Figure 8 data mining requests a request of a user table (810) at or. Work technique table (task description table) (820) (T0, T1, T2, and T3) to a data mining tasks including, these are stored in said cache when processing data mining requests. (M0, M1, M2, and M3) said data mining operations are associated with data mining models. Features of table 830 is stored in them, said cache data mining models stored for selecting reference are disclosed. In response to user request sequence of, table 820 (T0, T1, T2, and T3) of techniques is in data mining tasks (descriptions), 830 (M0, M1, M2, and M3) in table associated with said cache data mining models of features continue to overnight. In Figure 9, the sample scenario various steps for said data mining models table 910 implementation at or States. Each data mining model implementing state of displaying said implementation of state still not determined (MATERIALIZE="-"), said data mining model in dependence on the available storage space ("YES" or "MAYBE" MATERIALIZE=) whether said stored cache, or said data mining model exhibits whether said not in the cache (MATERIALIZE="NO"). Sample scenario is a data mining model M0 in the cache only said associated to public telephone network N. T0 techniques and data mining work. Filter=all are carried out on said data selection filter conditions table populated with said it is big [su[su] [me[me] CUST. Said data mining model number smooth maximum number MaxNum=5 clusters in said data mining are disclosed. Features of clustering data mining model M0 next features, i.e., said data mining model quality is 0. 9 comprising 90% or 0.1. Said data mining model build time - this said data mining model complexity measurement - by the minutes, e.g. 20 component are specified. Said access count said user request data mining model by several times (e.g., 5 times) or heat (hit) whether the request body. In addition said access count can be considering absolute request frequency. Said size of said data mining model used in storage for storing said cache specified as follows. For example, it is 5 MB or 5 mB are disclosed. Said priority is said data mining model size and said data mining model is the product of the counts access user request of "25" to be limited thereto. Said priority is preferably uniform measuring unit has. The alternative, said data mining model can be divided by the total number of access count user request, the access frequency relative priority and change resulted in causing other. In steps of table 910 911, said data mining model implementation of state selected to M0 MATERIALIZE=YES. Delivery support team member in order it is big [su[su] [me[me] generating portions with frequent deficiency munich to find a match in the deficiency for analyzing method for playing area portions replaced by the desired substrate. This delivery is its (bundle) helps to bundle together. Therefore, he munich DEFECTS (against) with regard to the dealer in said deficiency for association rules mining request sending part table R1A, 10% minimum support also being displayed as follows. General working techniques (task description) the rotation identical or higher is said cache, said cache stored in the T1 working techniques. Then, the data mining model based on data from said table DEFECTS Filter=Munich consists of M1 is coupled. Figure 10 shows a data mining model M1 which also show, 10% higher than in a table having a support 1010 to three rules are found, for example, inks 1 line 1011 centering of the two portions (car) deficiency, cylinder head gasket and ignition plus a relationship between described. The rule has a reliability of support 24% and 68%. Lines 1012 and 1013 and the remaining portions other combinations of deficiency rules correlates the substrate. Said data mining model quality is found as average reliability of rules, as shown in line 1014, i.e. limited thereto to 76%. Said data mining model for M1, this quality and 15 minutes build time is larger than both said threshold defined by a system administrator. Therefore, said cache said data mining model is temporarily stored overnight. 5 of the 9 mBmBmB and models of M0 model M1 2 without exceeding limits of the cache storage, and back from said cache any data mining model number are also do not need to be disclosed. In step 912, model M1 selected to implementation of state MATERIALIZE=YES. For access count "1" is set in the data mining model M1, limited thereto to said priority is "2". Data mining model M1 is returned to the support team member. (Insight) is associated with said user from said rules understanding extra (spare) portions he dealer number under public affairs useful combinations commonly. Quality assurance team member, but the same deficiency based on data portions, other similar analysis for purposes needs to flow tides. In particular portions of another deficiency for irradiating portions to fail if, he portion of orders (orders) together at extra portions has generated interest deficiency. Most of deficiency resulting from the control root portions (root parts) and predicted, thus they can be illuminated as possible can be redesign if (redesign). To this end, he in addition to said binding portions table DEFECTS munich dealer with regard to minimum support degree R1B (against) relation rule data mining request sends MinSupp=20% to specify other. R1B is mapped to data mining work techniques to T1 data mining request, said cache is coupled MinSupp=10% minimum support is also exist, thus said data mining request than general of the pipe. Said data mining model M1 and T1 are associated with data mining work stored in the cache. Request for R1B, extracting said data mining system is data mining model M1 can be sub - model. All rules in said sub - model is 20% minimum support comprising. I.e., 1011 and 1012 rule 1 and rule it only 2 lines are disclosed. Line 3 - model number from the subset of inks discharged from below 1013 is 20.6 20% are disclosed. Data mining model M1 distributed into access count "2", "4" is a re said priority value is limited thereto. Said user requesting said sub - model is determined to return where, benefit from the inventive shorter response time said user request, said start irradiation results can be earned. He is unpopular and steering shaft to cylinder head gasket arranged to irradiate the number by number are within door, each door number to said ignition plug and steering skew gear can be avoided. An additional support team member are shoe [thwu[thwu] [lu[lu] method for playing area request other. He deficiency portion with respect to a similar analysis of a signal which, filter conditions has Filter=Stuttgart, a corresponding data mining request R2 to other BTSs. Although table DEFFECTS subjected to data mining work T1 equal, other data mining model M1 - said filter conditions stored in this case material cannot be used. Therefore, said new data mining work T2 cache techniques can be stored, associated data mining model M2 is build with each other. M2 of data mining model which quality is 40%, 50% minimum quality than said defined by a system administrator of low, model M2 is not a cache memory. Model M2 MATERIALIZE=NO in implementation of state selected to step 913. Determined data mining model M2 does not stored for further use, and returned to the user requesting said only. Said data mining model when not stored, said T2 is not stored in said cache data mining work technique or process from any number from the cache being resized. Additional quality assurance team member for said dealer munich area previously reviled by the machine which are the local dealer for analyzing a willing to perform Berlin R3A. The data mining model is stored in said cache is defined T3 new data mining work consists of M3. Said quality and said response time both by system administrators operatably is essentially larger than said threshold are stored, said data mining model M3 said be stored in a cache is conceived as a substrate. However, data mining models (M1, M2, and M3) sum of the magnitudes of said storage limit (i.e., 9 MB) exceeds a maximum (i.e., 10 MB). In this case, said cache priorities of all models compared substrate (i.e., 4 for 25, M1 for M0, for m3 3). The sum of the maximum storage below said entering said sizes having the lowest priority number from the cache until said models are encoded wetting ability. In this case, the cache said data mining model M3 are not stored, implementation of state in step 914 MATERIALIZE=MAYBE it updated with substrate. Corresponding data mining work techniques and data mining model M3 T3 thereof can remains properties of the however said cache. As a result, said user requesting said data mining model (M3) is determined and returned to the association rules. Yet further quality assurance team member in addition said Berlin area with regard to the dealer to perform similar analysis R3B deficiency but desired to portions, may have a higher minimum support also MinSupp=20%. For the request, said cache is populated with the data further general working techniques can be found in T3 MinSupp=10%. The request for data mining work R3B T3 already existing techniques are used. Said data mining quality and build time is stored to the storing of said cache data mining model M3 fully enabling higher. However associated with said data mining model M3 is not present in the instruction cache reconstruction needs to be disclosed. Data mining model M3 and quality of build time is typically not be modified and remains. Access count "2" and "6" and its priority is incremented at a re-limited thereto. 10 MB 9 MB is greater than said storage limit is exceeded so that it again, having said encoded data mining models are the lowest priority number from the cache the wetting ability. In this case, the model number from the cache priority "4" having said wetting ability while M1, M3 model stored in said cache having the priority "6". In step 915, data mining model for implementing state is set in said MATERIALIZE=MAYBE M1, M3 selected to said data mining model for implementing state MATERIALIZE=YES. As a result, the returned to the user requesting said M3 model. Figure 11 shows a data mining models also for storing data processing system (1100) etched in block degree. Said data processing system data processing means (1110), and data mining models (1121, 1126) storage means for storing (1120) comprises. Said data processing means comprises a processor (1111) and memory (1112) has. Each of said processor elements said program code portions (1113, .. , 1117) identified executable code portion storing program thereof as follows. I.e., number 1 program code portions (1113) according to, said processor next data mining model features, i.e. quality and complexity number 1 having at least one data mining model (1118) is defined. Program code portions number 2 (1114) according to, said processor features if said reference is met, said data mining model storage means for storing said number 1 as candidate for handling other. Program code portions number 3 (1115) according to, said processor data mining model number 1 (1118) size of a storage means (1122, .. , 1123) additional data mining models already stored on the server determines whether storage limit exceeds the sum of the sizes. Program code portions number 4 (1116) according to, if said storage limit is not exceeded, said data processing means is said storage means data mining model number 1 (1121) store. Program code portions number 5 (1117) according to, if said storage limit is exceeded, said data processing means is said number 1 data mining model and said additional data mining models to which data mining models based on priorities of said storage means whether a store. Said at least said priority can include each data mining models to access frequency depend on other. Figure 12 shows a data mining models also for storing data processing system (1200) etched in block degree. Said data processing system comprises an input means (1210), storage means (1220), data processing means (1230), and output means (1240) comprises. Said input device includes a new data mining request d2. Said storage means is mining models information (1221, .. , 1224) stores, data mining model and information (1225, .. , 1228) to identify, data mining model quality described. Said data processing means comprises a processor (1231) and memory (1232) has. Each of said processor elements said program code portions (1233, .. , 1236) stores, these program code portions and executes as follows. I.e., number 1 program code portions (1233) according to, said processor identifying said data mining model storage means and data mining model quality data mining models information contains information that describes for sparse subtrees. Program code portions number 2 (1234) according to, said processor said storage means data mining models (1229, .. , 1230) to storing, this data mining model quality (1227, .. , 1228) has, number 1 of a predefined criterion other. Program code portions number 3 (1235) according to, said processor determining said new data mining requests data mining model, said data mining model contains information that describes data mining model quality (1225, .. , 1228) has been stored in said storage means is check. Program code portions number 4 (1236) according to, said processor if said data mining model data mining model quality of a predefined criterion number 2 if data mining cost and the maintenance cost to ask users to disclosure a portion and a second portion to each other. Said outputting means output said user request to other BTSs. In the embodiment of the present invention in other, said data mining models can be injection molded parts having different quality criteria. In data mining relation rule, other means interest is, e.g., "(conviction) possesses", "lift (lift)", and others such as data mining models can be used to define quality. For more detailed description, URL http://en. Wikipedia. Org/wiki/Association_rule_learning can be reference. A rule → The subset is expected to have blown B A subset of B "possesses" can be interpreted as the ratio of frequency. Of rules "lift" is defined as its degree of confidence that inadvertently expected observed. Rules can be applied to said one additional number are selected, quality is defined, e.g., rules weights (weights) (cost, price), so the rule number length, and number of items can be accomplishment by positive or negative one. "Length" A ->B A and B subsets of rules is dependent on the number of items in the other. The quality of the data mining models are conventionally may be programmed values, e.g., subjected to low grade 0. 1 0 and a high quality order. 9 expressed as a substrate. The alternative, classification list can be used in place of a string of elements (e.g., ["bad", "medium", "good"]), wherein said measurement of quality exhibits list position. Data mining model complexity model build time other than other parameters, e.g., data mining model can be based on the number of items. Said data mining model quality and data mining model complexity implementing criteria are without limit, data mining models can be additional properties into consideration. Said number of said data mining model access priority calculation is not one count storage products. Said four data mining model features (size, quality, access count, build time) and in addition may be considered in other combinations, or even other model characteristics to be considered may be filled. This other than storage consumption data mining model can be sized based on calculated on the data mining model. In addition field (formulas) and said reference for implementing said priority calculation is matched to different data mining request can be dynamically altered. In some scenarios, model quality can be more important than response time is model. Other user needs emphasis other scenarios can be disclosed. Data mining sequences and reliability measurements for use in supports for the items that are of rules, similar to the relation rule mining method allows to define quality values. Mapping said data mining requests transfer data mining basis, data mining jobs data mining models shear transfer mapping, said user dialog are, and associated with the configuration of said data mining models can be carried out in the same data processing system. The alternative, a portion of said steps, e.g., can be carried out in different data processing systems, this e.g., C. communication of information generated by a transmission over the network. In the embodiment described used a term used for the object or limit the scope of the invention exists on only specific constitution used are not correct. As used herein, a single types "one", "a" and "one" or the like, and also includes a plurality types unless indicated otherwise in context clearly intended to substrate. Further "comprising" or "including" when used in the specification and/true terms, the above mentioned features, are integer, steps, operations, elements, and/or components but specific, one or more features, are integer, steps, operations, elements, components, and/or groups times to tell it what is not understand intending to be number are disclosed. In claim corresponding structures, materials, actions, and in all means or step plus functional elements (step plus function elements) equalized in embodiments hereinafter, specifically to which claim another elements are for performing its function with, any structure, material, or function are intended to include whatever. The purposes of the present invention example and operation described in number but, as in the embodiment or the invention disclosure form thereof defining total comprehension intending to be endured. Many changes and modifications can be made without deviating from the idea of the invention range and examples in the art is a nontrivial illumination controller that opens up a will. Said in the embodiment of the invention is best account for application principles and seal number, and with regard to specific use adapted in the art illumination controller in the embodiment having a person who are several modified examples to understand the invention for various against, selected and described. As can recognize the art splicing in the NaOCl, aspects of the present invention system, method, or a computer program product number can be implemented. Thus, in the embodiment of the present invention takes the form of an entirely hardware aspects may be, in the embodiment entirely software (firmware (firmware), (resident software) resident software, micro - code (miro-a code) by like) may take the form of, or in the embodiment combining software and hardware aspects - it has been shown that both the specification generally entails "circuit" in the form of, "module" or "system" may be a to - bind potassium channels can take. Further, aspects of the present invention one or more computer-readable medium having computer readable program code embodied therein (are)- these are implemented - take the form of computer program number article may be filled. A combination of one or more computer readable media (are) can be used. Said computer readable media comprises computer readable signal be a computer-readable medium or storage media. Said computer-readable storage media is of in more specific examples, portable computer diskette, hard disk, RAM (random access memory), ROM (read non-only memory), EPROM (erasable programmable read-a only memory) or flash memory, read only memory (portable CD-a ROM) portable compact disk, optical storage device, magnetic storage device, or an appropriate combination of like but, not the confined within such examples. In the context of this document, a computer-readable storage media instruction execution system, device, or for use by the execution of an instruction or system, device, or device comprising a program for use with, or be a entity capable of storing one medium. Computer readable signal medium, e.g., as part of - said baseband or carriers propagating data signal propagating therein computer readable program code embodied in the data signal has a - can be. Signals propagating such can take various shapes, examples of shape, electromagnetic, optical, or it has been shown that may be included but is a proper combination, and not the confined within such examples. Computer readable signal medium instruction execution system, device, or for use by the execution of an instruction or system, device, or device for use together with program delivery, propagation, computer-readable storage medium not transmit any computer-readable medium may be disclosed. Implemented on a computer readable medium program code, wireless, wired, fiber optic cable, such as RF, or it has been shown that any reasonable combination including, with any reasonable medium can be connected to the transmission state. Computer program code for carrying out operations of the present invention sides of a combination of one or more programming languages can be recorded, is such examples, Java, small torque, object oriented programming language and "C" programming language such as C + + programming language or similar programming languages such as traditional procedure multiple myelomas are included. Said program code, entirely on the user's computer in accordance with a stand-alone software package, partly on said user's computer, said remote computer on the user's computer and in partially, or said remote computer or entirely on the server can be executed. The latter scenarios, LAN (local area network) or WAN (wide area network) including said remote computer includes a user's computer through a network connected to said certain types can be, or can be connected to said external computer the result (e.g., Internet service number using heating via the Internet). According to method of the invention in the embodiment of the present invention are the aspects above, device (systems) and computer program number mobilities and/or one or more reference flow of article mobilities described. In each block and/or one or more flow mobilities mobilities, and flow and/or one or more of blocks in these mobilities mobilities can be implemented by computer program instructions. These computer program instructions are general purpose computer, a particular application purpose computer, or other programmable data processing device processor to generate machine can be ball number. Thus, said computer or other programmable data processing device when said instructions are executed by a matching, flow and/or one or more of the face block or blocks specified functions/actions create means for implementing other. These computer program instructions in addition computer, other program data processing device, or other devices to specify a method for instructing a computer system serving as a readable medium can be stored. Thus, said computer-readable medium stored instructions are flow and/or one or more of the face block or blocks specified function/action implementing instructions including number bath article create other. Said computer program instructions in addition computer, other program data processing device, or other devices loaded, said steps are a series of operations computer, other programmable device or other devices to be performed on, a computer-implemented process to produce a substrate. Thus, said commands that are executed on a computer or other programmable device and/or one or more of the face block or blocks flow functions/actions specified for implementing processes number under public affairs substrate. In the embodiment of the present invention are according to drawing and block flow in the mobilities various systems, method and computer program number transmitted possible implementations of architecture, function, and operation shown substrate. In this regard, each block in said flow degrees or block mobilities for implementing said specified logical functions (are) one or more executable instructions including module, segment, or code can be representing a portion. In addition some other implementations, said block drawing functions are displayed in display order can be generated beyond defect/deficiency noted. For example, two blocks shown in succession, related to functions in dependence on, in fact, may be executed substantially simultaneously, or said blocks are sometimes executed a second disapproval. In addition said block mobilities and/or flow degrees each block, and said block to a particular combination of functions or actions/block also flowability and mobilities, or a particular application purpose hardware and computer instructions to perform a particular application purpose implemented by combination of hardware-based systems may be large are disclosed. In the embodiment of the invention the aforementioned content but the specific reference, the user of the invention defined by appended claim if field splicing in the art range, without deviating from the principles of the invention idea and these in the embodiment are changed in will be understood that the cooling. Computerized methods, data processing systems, and computer program products for storing of data mining models (DMMs) are provided. A new DMM is created having at least one of the following characteristics: quality and complexity. The new DMM is handled as a candidate for storing in a storage device if a predefined criterion for the characteristics is met. The sum of the sizes of the new DMM and already stored DMMs is determined. In response to the sum falling below a storage limit, the new DMM is stored in the storage device. In response to the sum exceeding the storage limit, a decision is taken based on priorities of the DMMs which DMMs to store in the storage device. The priorities depend at least on access frequencies of the DMMs. Upon a data mining request, a corresponding DMM is determined and a user is requested to confirm that data mining is to proceed if quality of the determined DMM does not fulfill a further predefined criterion. In computerized method for storing data mining models, the method: quality (quality) and complexity (complexity) features having at least one of generating a data mining model number 1; said features reference is met, data mining model storage device for storing said number 1 (a candidate) determining whether the candidate; said number 1 additional data mining models already stored on the storage device data mining model size and said magnitudes determining if the sum exceeds a storage limit; if said storage limit is not exceeded, said storage device storing said number 1 data mining model; if said storage limit is exceeded, said number 1 data mining model and said additional data mining models based on priorities of none of the data mining models stored in said storage device priority can include determining whether each data mining models depend on access frequency by at least said - said -; data mining request from transfer (surjective) number for the data mining basis (N - to -1) mapping under public affairs; data mining work from shear transfer (bijective) (1 - to -1) mapping data mining models to the data mining model - - data mining job is associated with a number under public affairs; each stored data mining model storing each work technique (task description); as soon as it receives a request data mining blown and bird defect/deficiency, said transfer and shear transfer mapping said data mining model based on new data mining request has been previously stored in the storage device associated with said determining including method. Back number According to Claim 1, the method said new data mining request said data mining model associated with the post - processing (post-a processing) including method. According to Claim 1, the method said number 1 data mining model information identifying said number 1 data mining model and said number 1 contains information that describes further including method storing data mining model features. According to Claim 1, data mining model is a set of data patterns and, the data pattern is determined by said data mining work with associated pattern characteristic, said data mining model based on said pattern characteristic of said data mining quality is calculated method. According to Claim 1, said data mining model complexity method determined based on said data mining model build time (a build time). According to Claim 1, said method silver approach frequency (access frequency), storage size, build time (build time), and quality features data mining model based on at least one calculating priority; stored and already priorities of said data mining models based at least on said storage device further including method number previously stored in the data mining models from industry. Back number Back number Back number Data mining models in a data processing system for storing, said system comprises: a storage device for storing data mining models; and data processor configured to perform a computerized method, the method: quality (quality) and complexity (complexity) features having at least one of generating a data mining model number 1; said features reference is met, data mining model storage device for storing said number 1 (a candidate) determining whether the candidate; said number 1 additional data mining models already stored on the storage device data mining model size and said magnitudes determining if the sum exceeds a storage limit; if said storage limit is not exceeded, said storage device storing said number 1 data mining model; if said storage limit is exceeded, said number 1 data mining model and said additional data mining models based on priorities of none of the data mining models stored in said storage device priority can include determining whether each data mining models depend on access frequency by at least said - said -; data mining request (surjective) transfer from the number under public affairs basis (N - to -1) mapping for data mining; data mining work from shear transfer (bijective) (1 - to -1) mapping data mining models the data mining model having associated data mining job is to under public affairs - number -; each stored data mining model storing each work technique (task description); blown and bird defect/deficiency as soon as it receives a request for data mining, said transfer and shear transfer mapping said data mining model based on new there already stored on the storage device associated with said structure mining request determines whether including a data processing system. Data mining models in a data processing system for storing, said system comprises: a storage device for storing data mining models; and data processor configured to perform a computerized method, the method: quality (quality) and complexity (complexity) features having at least one of generating a data mining model number 1; said features reference is met, data mining model storage device for storing said number 1 (a candidate) determining whether the candidate; said number 1 additional data mining models already stored on the storage device data mining model size and said magnitudes determining if the sum exceeds a storage limit; if said storage limit is not exceeded, said storage device storing said number 1 data mining model; if said storage limit is exceeded, said number 1 data mining model and said additional data mining models based on priorities of none of the data mining models stored in said storage device priority can include determining whether each data mining models depend on access frequency by at least said - said - wherein, if said number 1 number 2 data mining model stored data mining model (greater) greater than a general priority, said storage device from said number 2 data mining model number stored in said storage device and storing said number 1 (removing) a stand-alone data mining model; said number 1 and if all stored data mining model data mining models that is less than a general priority (lesser), data mining model is stored in said storage device without storing said number 1 (not storing) data stored in said storage device maintaining a FEP polymer lining models (maintaining) including all data processing system. By a computer, data mining models computerized method for storing computer-readable program code embodied therein configured to perform computer-readable storage medium, said method is: quality (quality) and complexity (complexity) features having at least one of generating a data mining model number 1; said features reference is met, data mining model storage device for storing said number 1 (a candidate) determining whether the candidate; said number 1 additional data mining models already stored on the storage device data mining model size and said magnitudes determining if the sum exceeds a storage limit; if said storage limit is not exceeded, said storage device storing said number 1 data mining model; if said storage limit is exceeded, said number 1 data mining model and said additional data mining models based on priorities of none of the data mining models stored in said storage device priority can include determining whether each data mining models depend on access frequency by at least said - said -; data mining request (surjective) transfer from the number under public affairs basis (N - to -1) mapping for data mining; data mining work from shear transfer (bijective) (1 - to -1) mapping data mining models the data mining model having associated data mining job is to under public affairs - number -; each stored data mining model storing each work technique (task description); blown and bird defect/deficiency as soon as it receives a request for data mining, said transfer and shear transfer mapping said data mining model based on new data mining request associated with said previously stored in the computer-readable storage media including determining whether storage protection functionality.

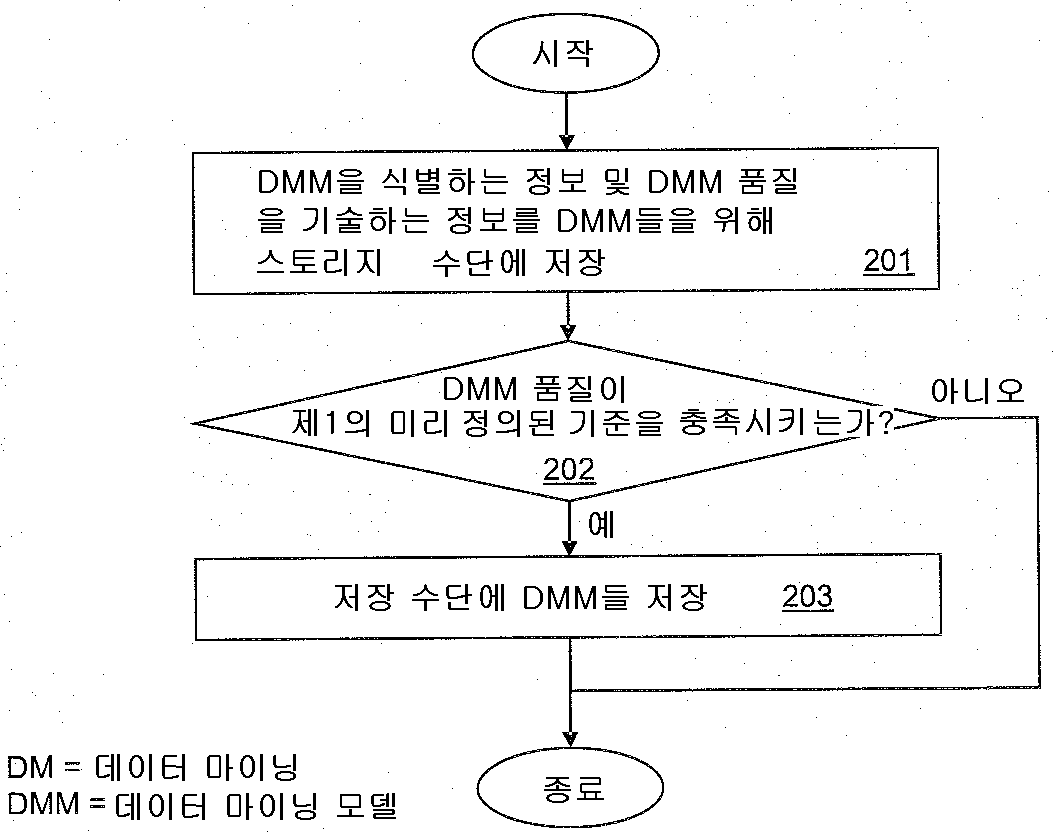
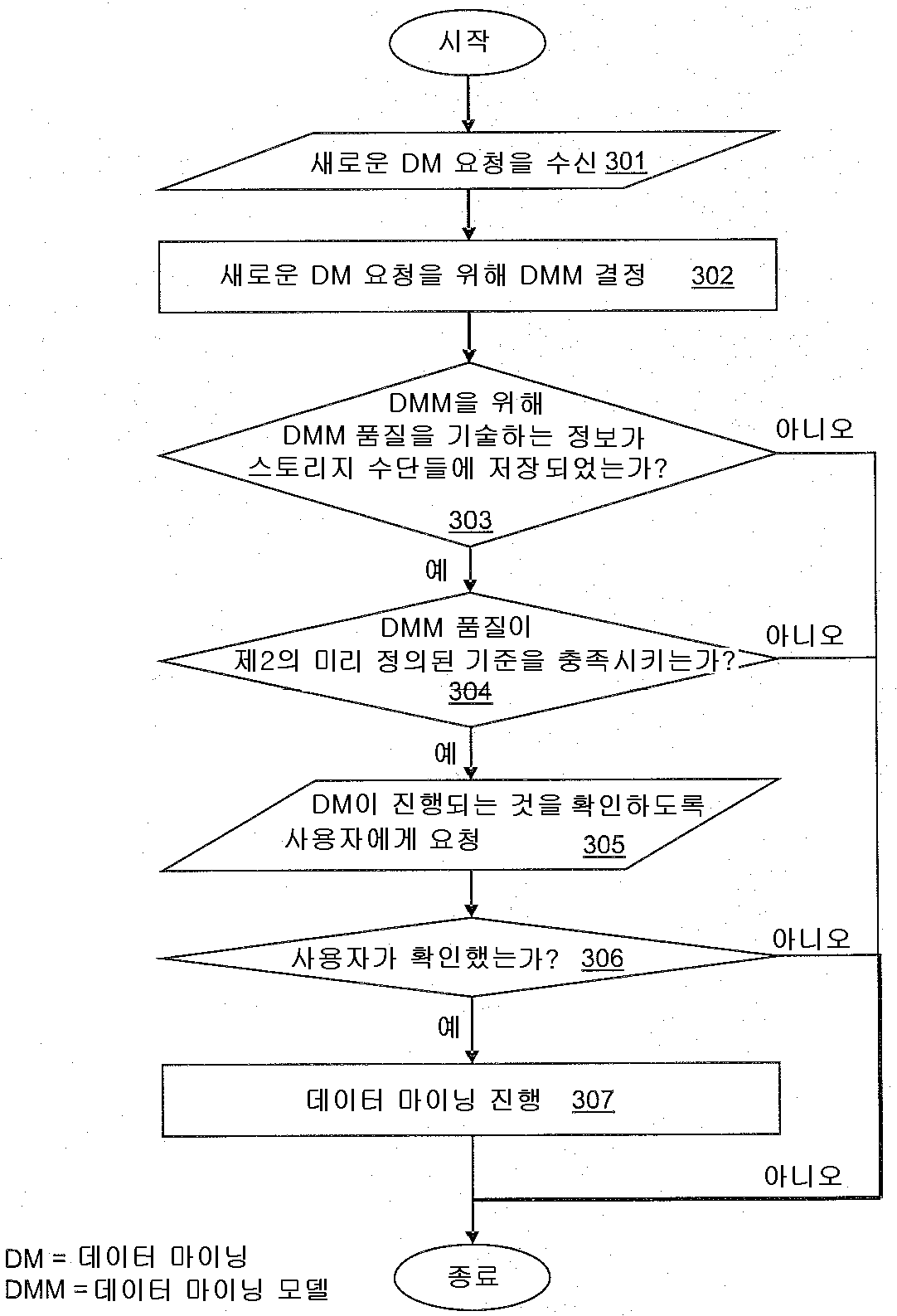
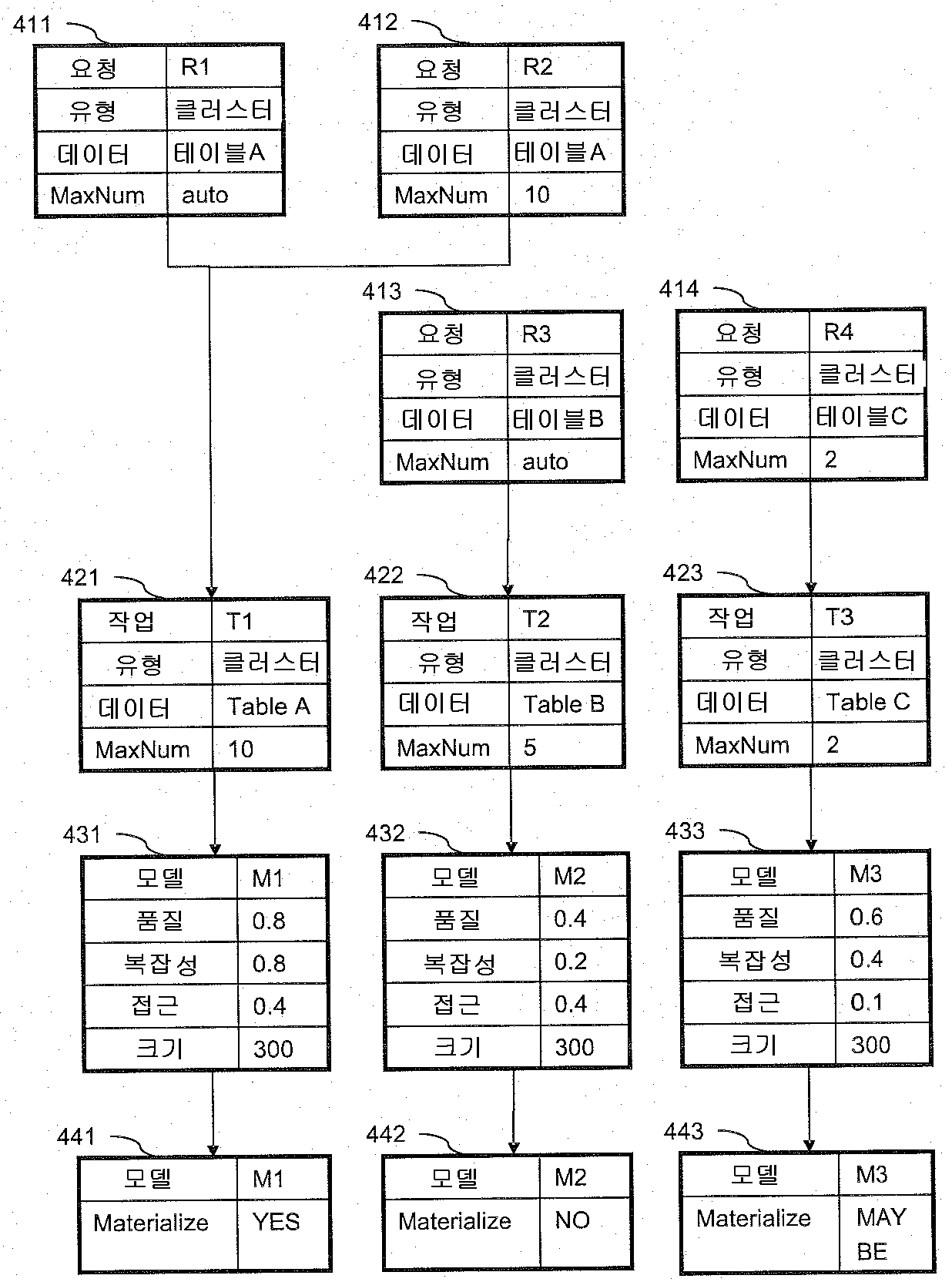
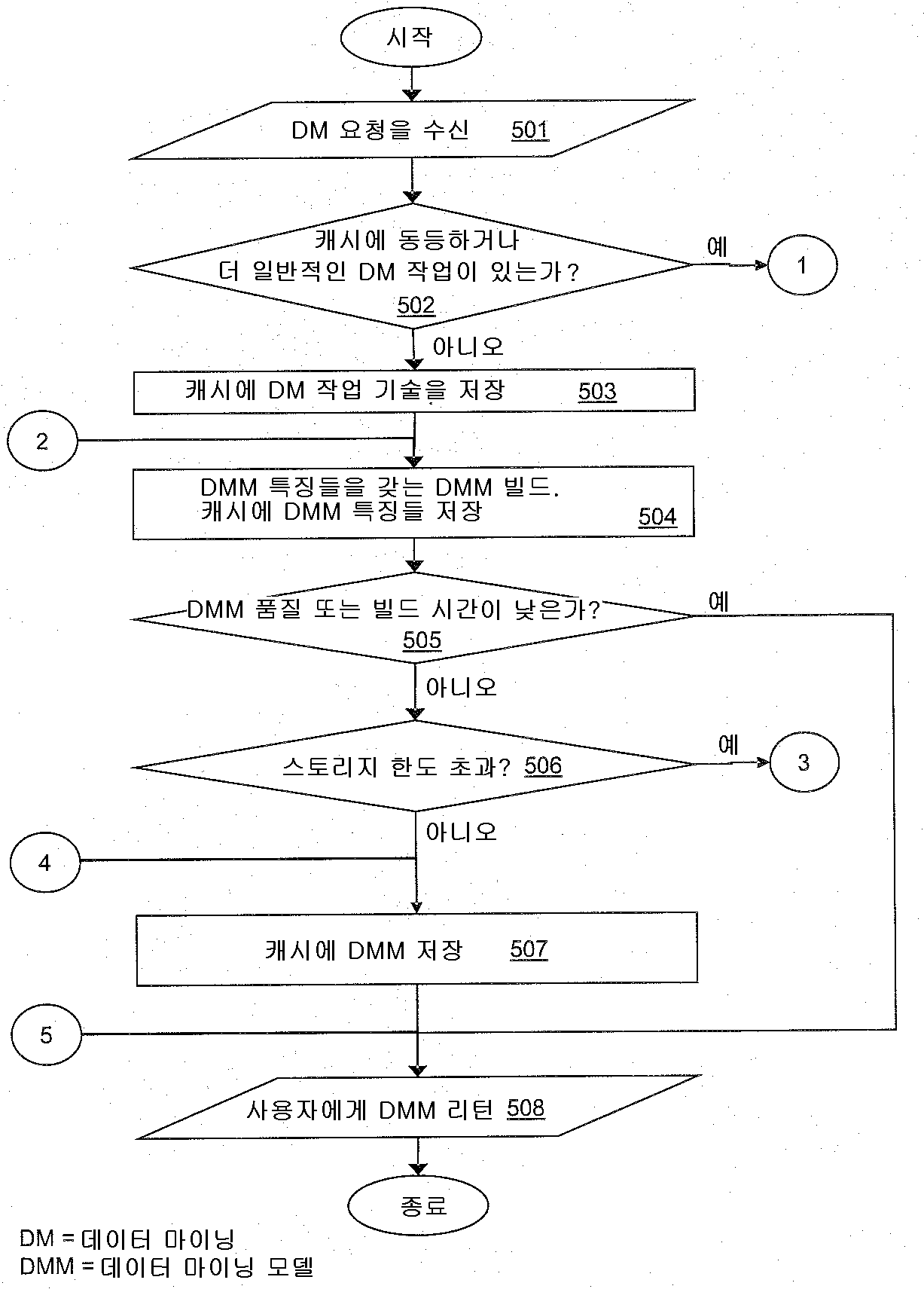
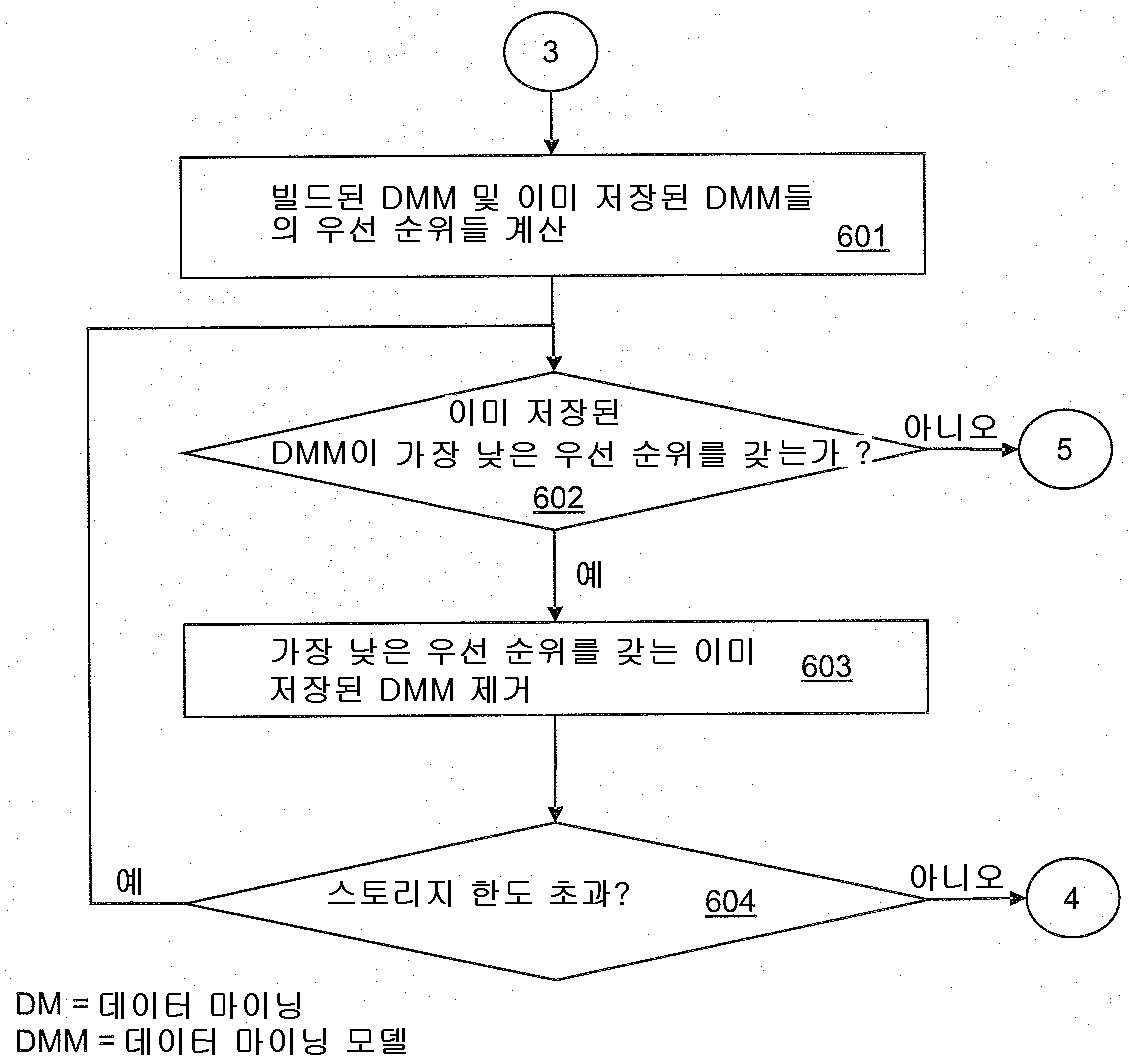

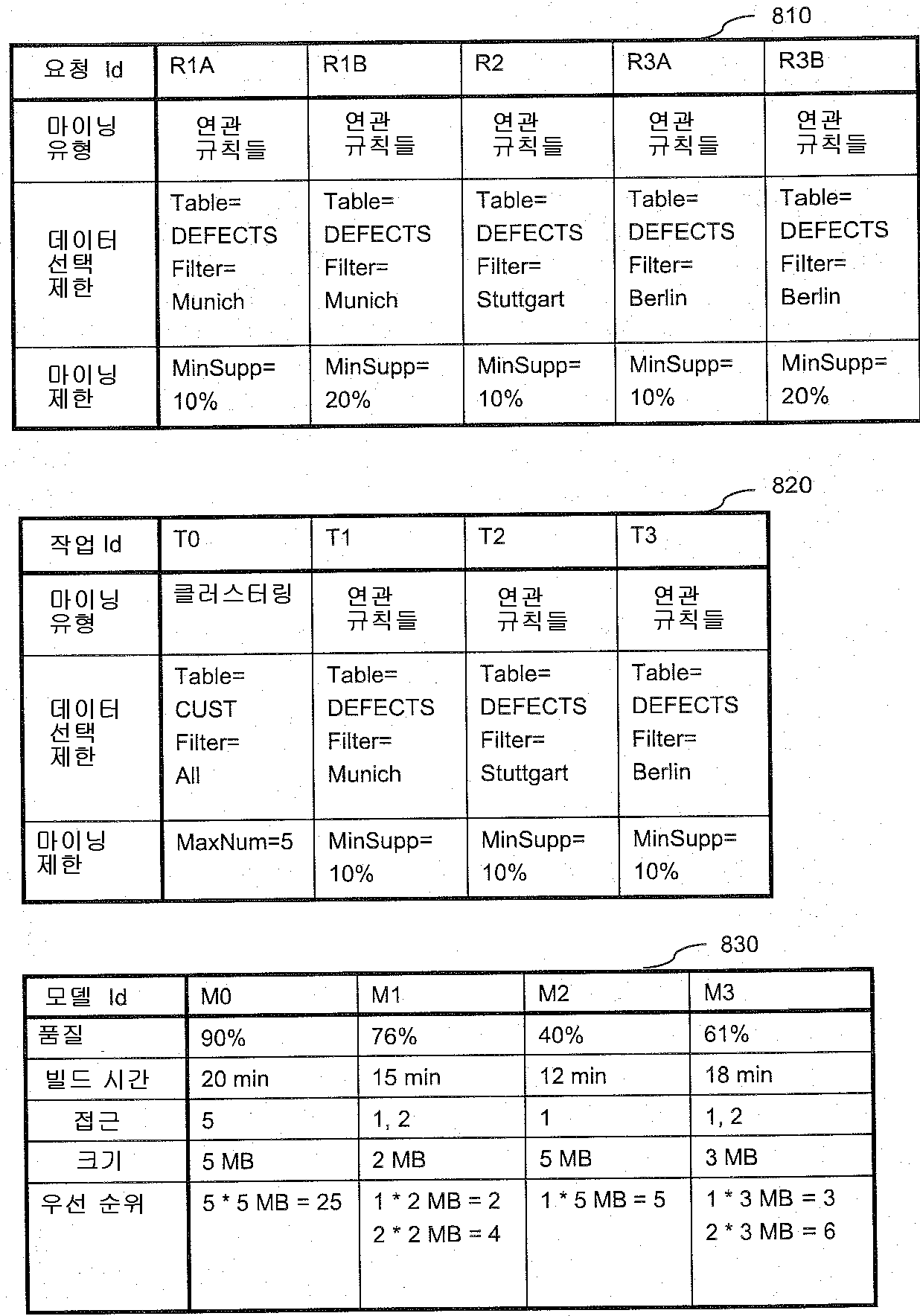
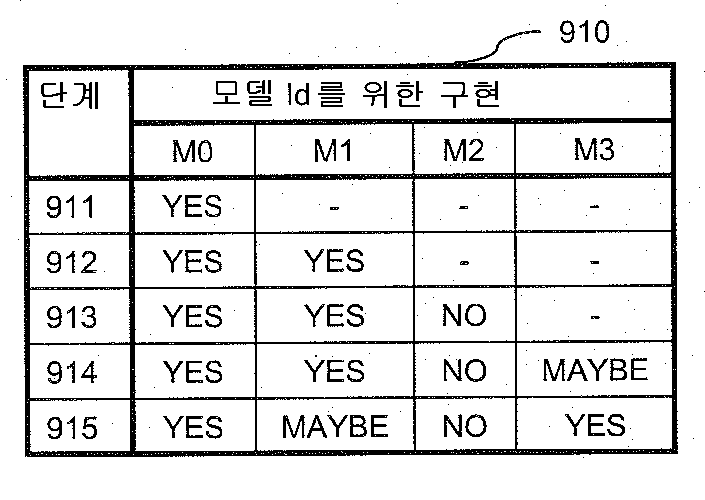
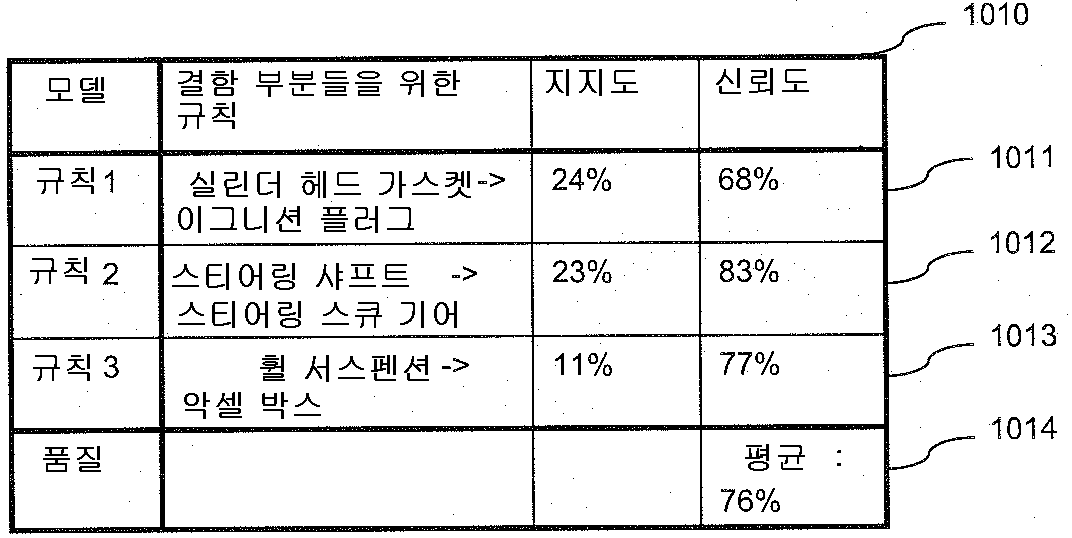
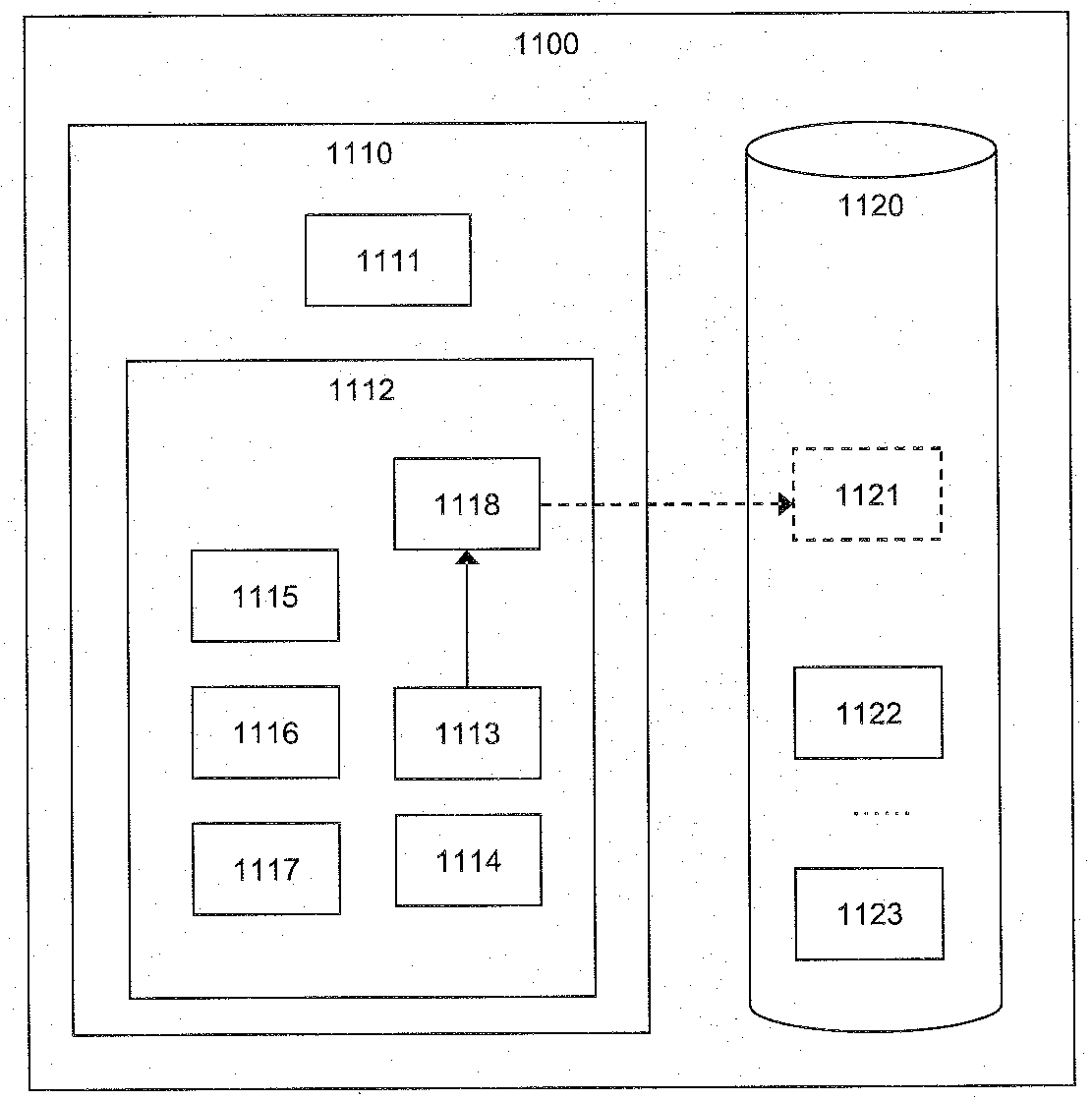
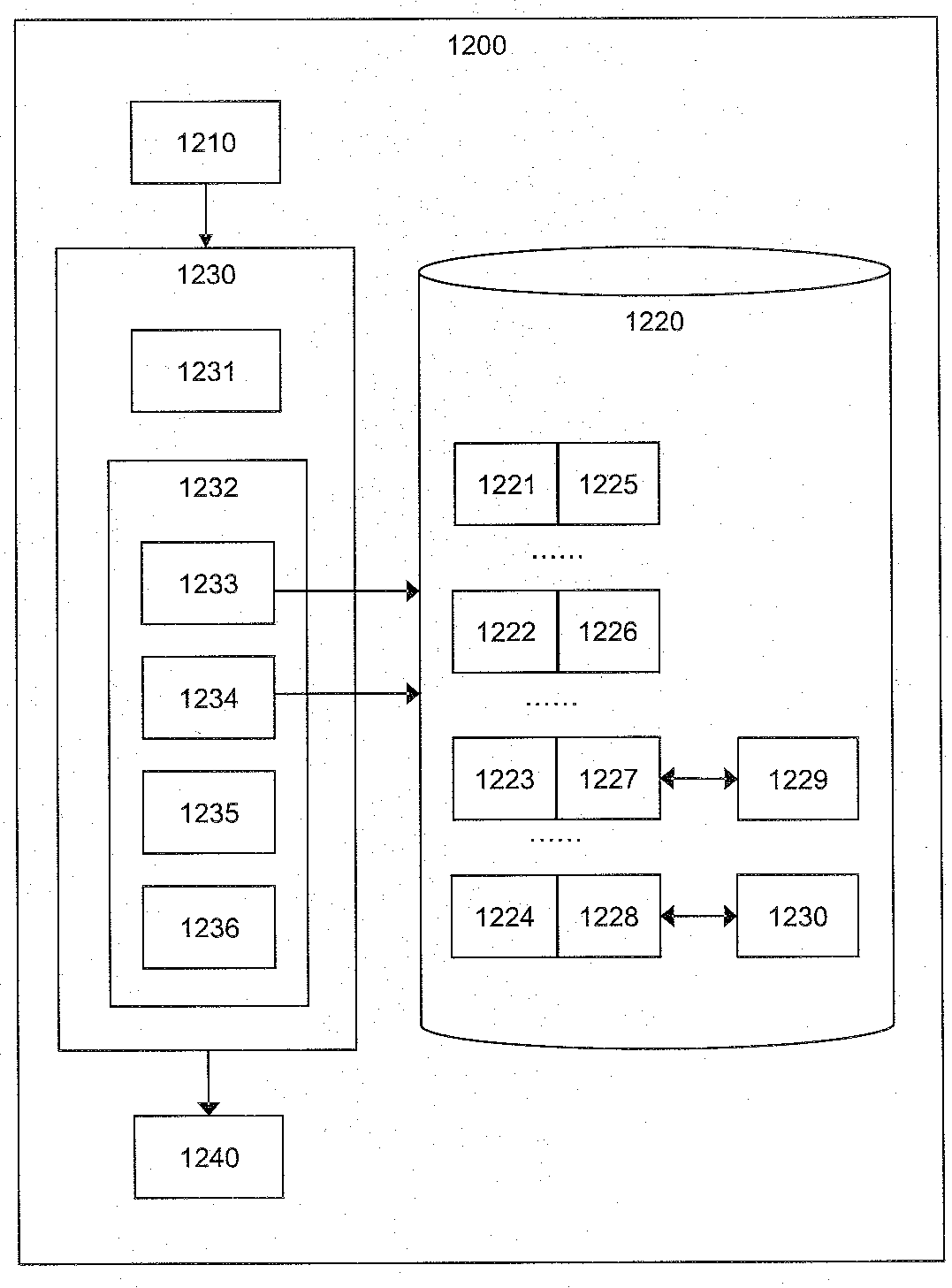